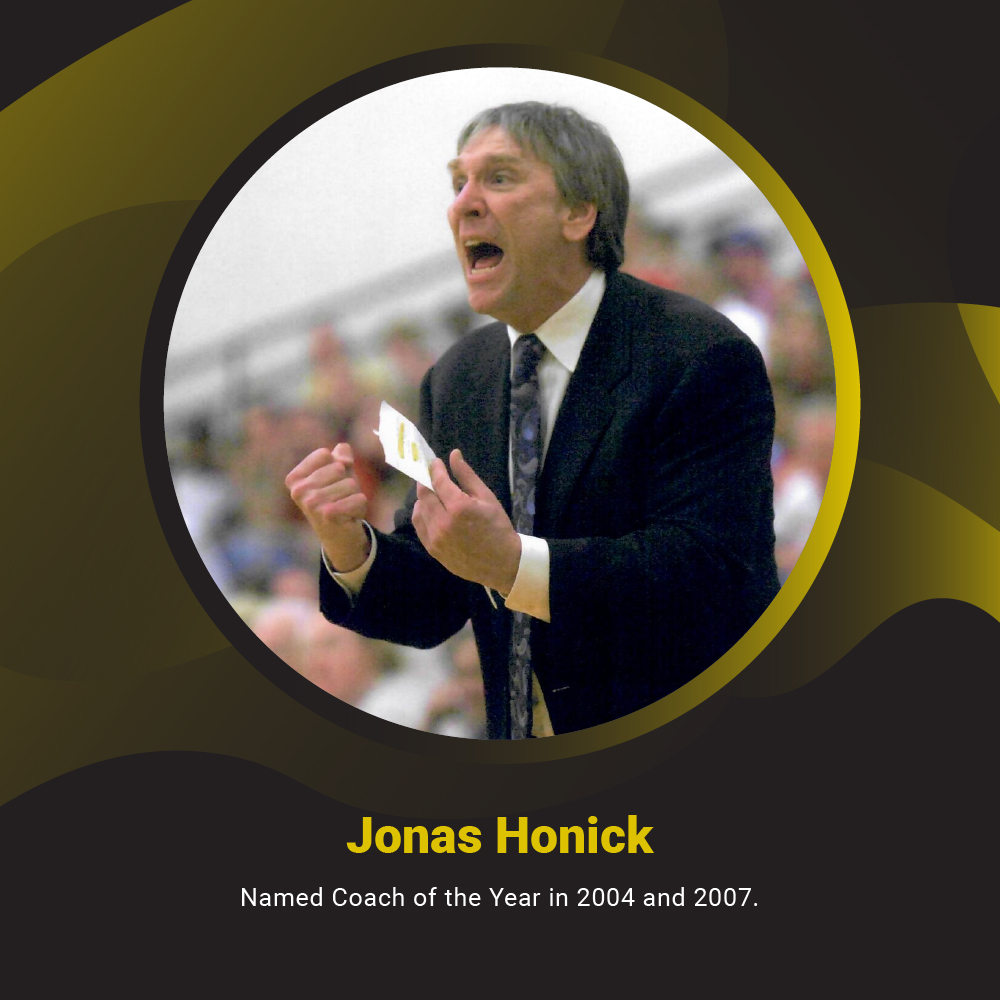
In the past decade, analytics has become an integral part of modern basketball, fundamentally changing how teams, players, and coaches approach the game. Gone are the days when basketball decisions were made solely based on gut feelings and traditional statistics like points, rebounds, and assists. Today, advanced data and sophisticated analytical models play a crucial role in decision-making processes both on and off the court. From player evaluations to game strategies, analytics has empowered teams to make more informed, precise, and efficient choices.
The Rise of Advanced Metrics in Basketball
Basketball has always been a sport of numbers, but the kind of numbers being tracked has evolved significantly. Traditional stats, such as points, rebounds, and field goal percentage, still matter, but advanced metrics have taken center stage. Terms like Player Efficiency Rating (PER), True Shooting Percentage (TS%), and Win Shares (WS) are now commonly used by front offices, coaching staff, and even fans.
PER, developed by ESPN’s John Hollinger, is an all-encompassing statistic that attempts to boil a player’s overall contribution down to one number. While traditional stats focus on counting achievements, PER goes deeper by accounting for a player’s efficiency. Actual Shooting Percentage incorporates a player’s free throws and three-pointers into their shooting efficiency, while Win Shares attempt to quantify how many wins a player contributes to his team.
Analytics allows teams to assess player value beyond the eye test and conventional statistics. For example, a player who scores a lot but does so inefficiently may contribute less to winning than a player who scores less but is highly efficient. Metrics like PER and TS% highlight these differences and enable teams to make smarter personnel decisions.
Shot Selection and Efficiency
One of the most significant ways analytics has impacted basketball is in shot selection and efficiency. Teams are increasingly relying on data to determine which types of shots yield the best results. The mid-range jumper, once a staple in the NBA, has fallen out of favor due to analytics. Teams now prioritize three-point shots and attempts near the rim, as these are statistically the most efficient ways to score.
Three-point shooting, in particular, has become an essential part of the modern game. Teams like the Golden State Warriors revolutionized basketball by using analytics to build a roster focused on spacing the floor and taking a high volume of three-point shots. The Warriors’ success, driven by sharpshooters like Stephen Curry and Klay Thompson, demonstrated the power of analytics in shaping offensive strategies. The idea is simple: even though three-pointers are more complicated to make, they are worth more points, making them a more efficient choice over time.
On the defensive side, analytics helps teams understand which areas of the floor they should prioritize protecting. Defenders are often instructed to run shooters off the three-point line and contest shots at the rim while allowing mid-range shots, which are statistically less efficient.
Player Health and Load Management
Analytics isn’t merely about what happens on the court but also revolutionizing how teams manage their players off the court. Player health and conditioning have always been critical factors in team success, but today’s teams use data to make more informed decisions about player workloads and recovery.
Load management, once a rare practice, is now standard in the NBA. Teams monitor players’ minuteplayers’sityminute player strength levels and physical condition throughout the season to prevent injuries and ensure peak performance during the playoffs. Advanced wearable technology tracks things like heart rate, distance traveled, and even biomechanics, helping teams optimize recovery times and reduce the risk of injury.
The Toronto Raptors’ use of the engagement with superstar Kawhi Leonard during their 2019 championship run is a prime example of how analytics can help teams manage player health. Leonard played in only 60 regular-season games to preserve his body for the playoffs, and the strategy paid off as he led the Raptors to their first NBA title.
Data-Driven Coaching Decisions
Analytics has also influenced how coaches make in-game decisions. From substitutions to play-calling, coaches now have access to data that helps them optimize game strategies in real-time. Lineup optimization is a significant area in which analytics has made an impact. Coaches can use data to identify which player combinations perform best together, both offensively and defensively. This has led to more effective rotation patterns and better matchups against opponents.
Timeouts, late-game situations, and even fouling strategies are now informed by analytics. For instance, the decision of whether or not to intentionally foul a poor free-throw shooter late in the game can be backed by data on how likely that player is to miss crucial free throws. Similarly, coaches can use data to decide whether to go for a quick two-point shot or a three-pointer when trailing by a specific margin late in the game.
Draft and Player Acquisition
NBA front offices are increasingly using analytics to guide their draft picks and free-agent acquisitions. Scouting, which was once primarily an art form based on observation, is now a blend of art and science. Teams have developed proprietary models that incorporate college and international player data to project how well a player will transition to the NBA.
For example, data might reveal that a college player who excels at getting to the free-throw line is likely to continue drawing fouls in the NBA, making him a valuable asset. Advanced data also helps teams identify “diamonds in the rough” players who may not have eye-popping traditional stats but possess skills that contribute to winning.
The Future of Analytics in Basketball
As technology continues to advance, so too will the role of analytics in basketball. Artificial intelligence, machine learning, and even more sophisticated tracking systems will further enhance teams’ ability to analyze and predict player performance, injury risks, and optimal strategies.
One potential area for future growth is real-time predictive analytics, which could provide coaches with actionable insights during the game itself. For example, a coach might receive data suggesting that a particular lineup is performing poorly on defense and make an immediate substitution.
Analytics has already transformed the way basketball is played, coached, and managed. As teams continue to innovate and explore new ways to utilize data, the sport will continue to evolve, creating a more efficient, strategic, and exciting game for players and fans alike.